AI/ML - PHY - Rx Blocks
Traditionally, AI's application within the RX chain has been segmented, focusing on optimizing individual components. However, the advent of fully convolutional deep learning models, like DeepRx, proposes a transformative approachapplying AI across the entire (or multiple component in a single chunk) RX chain. This method not only streamlines the process but also significantly enhances performance by leveraging AI's ability to learn and adapt to complex signal environments. This holistic
application of AI in the RX chain could redefine the boundaries of wireless communication efficiency and reliability.
An example is illustrated as below. In this example, an AI (DeepRx system) performs channel estimation, demapping, and equalization within the signal processing chain, replacing traditional receiver functions with AI-based methods.
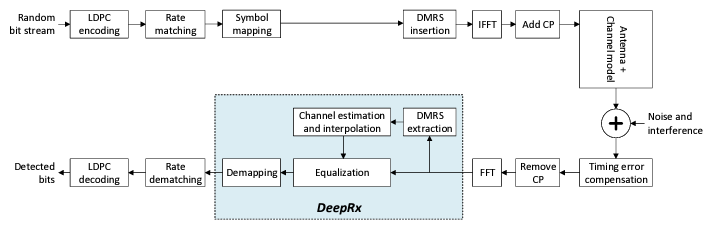
Image Source : DeepRx : Fully Convolutional Deep Learning Receiver
Some of advantage of applying AI model to replace entire or large blocks of Rx chain comparing to applying to a single process (e.g, application to channel estimation only) can be listed as below :
- Unified Architecture: A larger AI block learns to process the signal in a unified manner, potentially capturing complex interdependencies between different stages that individual blocks might miss.
- End-to-End Learning: The model can learn to directly convert the received signal into bit streams, which might allow it to find patterns and optimizations that modular designs don't capture.
- Data-Driven Approach: Using the original bit stream as a label for the AI's output allows for precise and straightforward supervision, making the AI's learning process more aligned with actual operational conditions.
- Adaptability: A broad AI model can flexibly adapt to a range of signal distortions and variations, which would require multiple specialized models if the RX chain were segmented.
- Simplified Training Pipeline: Since the input and output of the AI model are closely related, the training process becomes more streamlined, potentially leading to a shorter development cycle and less complex training procedures.
- Reduced Manual Tuning: With AI handling a larger block, there's less need for manual adjustment of individual components, which can be labor-intensive and requires expert knowledge.
- Efficient Implementation: A single, large AI block might use computational resources more efficiently than several smaller blocks, reducing redundancy and possibly the overall computational load.
Reference
|
|