MDA
Management Data Analytics (MDA) is key to modern network management. It uses data to improve network operations. Instead of reacting to problems, MDA predicts them. It analyzes large amounts of network data and this analysis provides valuable insights. These insights help optimize network performance and they also improve resource allocation. MDA enhances service quality. It automates management processes. Operators use MDA to make informed decisions.
They can
anticipate issues and address them proactively. This leads to a better user experience. MDA covers many areas, like congestion management and demand prediction. It also dynamically adjusts network parameters.
MDA collects data from various network elements, analyzes this data to gain insights into network behavior and performance, and then uses these insights to inform network operations and optimization. This process involves receiving diverse data inputs, applying analytical functions to identify trends and anomalies, generating reports and actionable outputs, and storing both raw data and analysis results for future use. Ultimately, MDA aims to automate and enhance network management
through data-driven decision-making.
Different parts of the network send data. These parts are called NFs, MnFs, and AFs. This data goes into the Management Data Analytics Function. This function analyzes the data. It also uses old data and reports. These are kept in the Data Repository. The analysis creates new reports. These reports go back to the NFs, MnFs, and AFs. This helps them work better. The Data Repository stores all the data and reports. So, the picture shows a
cycle of data analysis and improvement.
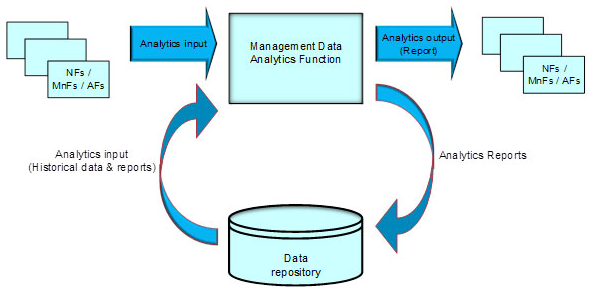
Image Source : Management Data Analytics (MDA)
Overall workflow of the MDA functionality can be summarized as follows
- Data flows into the MDAF from network components as analytics input.
- The MDAF processes the data using advanced analytics techniques and stores it in the data repository for future reference.
- Insights and recommendations are generated as analytics outputs and sent back to the relevant network entities.
- The feedback mechanism ensures continual refinement of the system’s analytical capabilities.
More detailed breakdown and description are as follows :
- Analytics Input
- Sources: Data inputs are gathered from Network Functions (NFs), Management Network Functions (MnFs), and Application Functions (AFs). These are key elements within a 5G network ecosystem responsible for various operations like traffic management, resource allocation, and application support.
- Types of Data: The input includes real-time operational data, key performance indicators (KPIs), and historical reports, all of which are essential for comprehensive analysis.
- Management Data Analytics Function (MDAF)
- Core Analytics Engine: This central component processes the incoming data. It uses advanced algorithms, often powered by artificial intelligence (AI) and machine learning (ML), to derive actionable insights from the raw data.
- Functions: MDAF supports a variety of use cases, including anomaly detection, network optimization, predictive maintenance, and service assurance.
- Data Repository
- Purpose: Acts as a centralized storage system for both historical and real-time data. The repository ensures the availability of data for longitudinal analysis, trend identification, and machine learning model training.
- Feedback Loop: The historical data and previous analytics reports stored here are used to improve the accuracy and relevance of future analytics outputs. This creates a continuous improvement cycle.
- Analytics Output
- Deliverables: The processed insights are delivered back to the entities that provided the data (NFs, MnFs, and AFs) in the form of detailed analytics reports. These reports may contain predictions, recommendations, or alerts.
- Impact: These outputs enable automated or semi-automated decision-making for network management tasks like load balancing, fault detection, or capacity planning.
- Feedback Loop
- The architecture has a feedback loop where the analytics outputs and updated data are fed back into the system. This iterative process improves the precision of analytics and adapts to dynamic network conditions.
The overall framework of the Management Data Analytics (MDA) system demonstrates the flow of data and analytics within a modern telecommunications network. At its core, the framework operates as a bridge between the network's operational components and the entities that consume analytics insights for decision-making and optimization.
The process begins with analytics input data, originating from various network domains such as the radio access network (RAN), core network (CN), transport network, and operations and management (OAM) systems. Non-3GPP management systems can also contribute input data, highlighting the system's flexibility in integrating diverse sources of information. This data encompasses a wide range of metrics and events related to network performance, user behavior, and operational statuses.
Within the management system, the MDA framework processes this data. Specifically, the Management Data Analytics Function (MDAF) serves as the central analytics engine. It applies advanced algorithms to transform raw data into actionable insights, predictions, and reports. These processed outputs, collectively referred to as analytics reports, are then delivered to the relevant entities that consume this information for network management and optimization purposes.
The framework showcases a continuous interaction where data flows from operational systems to the analytics engine and back to the consuming entities. This closed-loop design supports intelligent, data-driven management of networks, ensuring better decision-making, automation, and service optimization in complex environments like 5G.
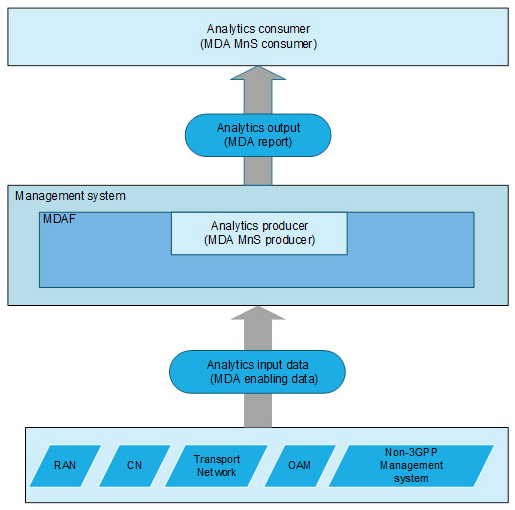
Image Source : Management Data Analytics (MDA)
Here’s a breakdown and description of the overall MDA framework. This detailed breakdown highlights the key components and their roles, demonstrating how the MDA framework facilitates intelligent and automated network management.
- Analytics Input Data:
- Sources: Data comes from various components, including the Radio Access Network (RAN), Core Network (CN), Transport Network, Operations and Management (OAM) systems, and even Non-3GPP management systems.
- Nature: The data includes network performance metrics, fault reports, operational statistics, and user behavior analytics, forming the foundation for analytics processing.
- Management System:
- Core Component: The Management Data Analytics Function (MDAF) is the central processing unit in the management system.
- Functionality: It acts as the analytics producer, taking in raw input data and applying machine learning or data processing algorithms to extract actionable insights.
- Outputs: These insights may include reports, alerts, trends, and predictions essential for network optimization.
- Analytics Output:
- Recipients: The processed data, referred to as analytics reports, is sent to analytics consumers. These consumers could be network operators, automation systems, or external management systems that use the insights to make decisions.
- Utility: The outputs help in optimizing network operations, troubleshooting issues, enhancing user experiences, and planning future capacity.
- Integration of Diverse Components:
- Interoperability: The framework integrates inputs from both 3GPP-compliant and non-3GPP systems, showcasing its flexibility.
- Comprehensive Data Utilization: By consolidating data from multiple domains, the framework ensures holistic analytics and actionable insights.
As an other way of presenting the MDA framework, we can refer to a diagram from TR 28.898 as show below.
This diagram provides an overview of the functional and service framework of Management Data Analytics (MDA). At its core, the MDA framework consists of interconnected entities, including the Management Data Analytics Service (MDAS) Producer, MDAS Consumer, and other components like the Management and Network Slice (MnS) Consumer and the Network Data Analytics Function (NWDAF). The MDAS Producer plays a pivotal role in analyzing data and generating actionable insights, which are then distributed
to MDAS Consumers for decision-making. This architecture allows for seamless integration of different producers and subscribers, ensuring efficient data flow and analytics sharing across the system. It reflects a robust and modular approach to managing complex networks by enabling analytics-driven automation and optimization in modern telecom ecosystems.
<TR 28.898 - Figure 4.2-1: Functional overview and service framework of MDA >
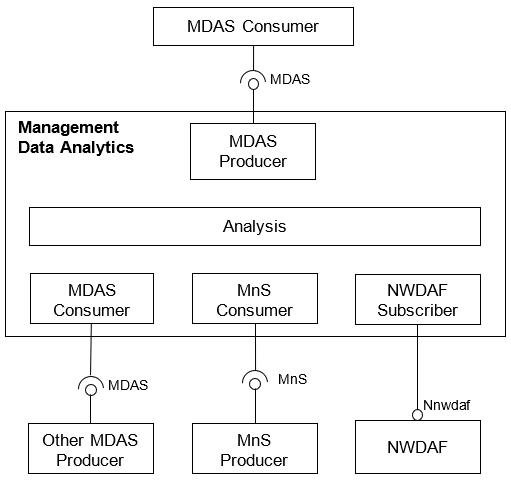
Here is the breakdown and description of the MDA functional and service framework as shown in the figure. This breakdown highlights a modular and interconnected system designed for the efficient generation, consumption, and sharing of analytics data. Each component plays a specific role in ensuring that the management system can leverage advanced analytics to optimize and automate complex telecom operations. The framework is designed to handle dynamic, data-driven decision-making in modern
5G networks and beyond.
- MDAS Consumer
- Role: The MDAS Consumer represents entities that utilize analytics data produced by the MDAS Producer.
- Functionality: It consumes analytics reports and insights for decision-making, automation, and optimization within the network management system.
- Examples: Network operators or systems responsible for dynamic resource allocation, fault management, or performance optimization.
- Management Data Analytics block
- MDAS Producer: The MDAS Producer is a core component that processes input data, performs analysis, and generates insights or reports. It acts as the analytics engine of the framework.
- Analysis Layer: This layer includes processing and analytics capabilities, leveraging machine learning or statistical methods to transform raw data into actionable outputs.
- Data Sources: The input data for analysis comes from various network domains and functions such as MnS Producers, NWDAF, or other MDAS Producers.
- MnS Consumer
- Role: The MnS Consumer is a specific type of consumer that focuses on analytics related to network slicing and service management.
- Purpose: It ensures that network slicing resources are efficiently allocated and managed based on analytics insights.
- NWDAF Subscriber
- Role: The Network Data Analytics Function (NWDAF) Subscriber integrates the framework with NWDAF, which is part of 5G system architecture and specializes in data analytics for the network.
- Purpose: NWDAF generates specific analytics that are utilized by the MDAS framework for enhanced functionality and insight generation.
- Other MDAS Producers
- Integration: The system is modular, allowing multiple MDAS Producers to interact and share data with one another.
- Purpose: Enables scalability and collaboration between different analytics producers within the ecosystem.
- Data Flow and Connectivity
- MDAS Interface: Facilitates communication between MDAS Producers and Consumers, ensuring that insights are delivered where they are needed.
- MnS and NWDAF Interfaces: Enable seamless integration with network slicing and NWDAF functionalities for specialized analytics and enhanced system interoperability.
Cross-domain Management Data Analytics (MDA) is an advanced capability within the 3GPP framework designed to enable seamless data analytics across multiple domains, such as the Core Network (CN), Radio Access Network (RAN), and other interconnected systems. The concept of cross-domain MDA emphasizes the interaction and collaboration of MDA functions (MDAF) across these domains to provide a holistic and unified approach to analytics-driven network management.
Cross-domain MDA acts as the bridge between various domain-specific analytics systems, integrating data from RAN, CN, and other network domains in order to create unified, actionable insights. By consuming and producing MnS across these domains, cross-domain MDA supports complex use cases that require holistic management and it ensures seamless collaboration, enhanced automation, and efficient network performance in the context of 5G and beyond.
Key Characteristics of Cross-Domain MDA
There are many different examples (use cases) of cross domain MDA but there are key characteristics of Cross-domain MDA which is applicable to most of the use cases as summarized below.
- Multiple MDA Instances:
- Depending on deployment needs, multiple MDA instances can be deployed across different domains, including CN, RAN, and other subdomains.
- These instances are designed to interact and exchange insights, creating a collaborative analytics ecosystem.
- Role of Cross-Domain MDA MnS Producer:
- In the 3GPP framework, the MDAF serving as a cross-domain MDA MnS producer consumes analytics and management services (MnS) provided by domain-specific MDA MnS producers (e.g., CN domain or RAN domain).
- This producer consolidates data from different domains, performs advanced analytics, and generates new analytics services (MDA MnS) that are consumed by 3GPP cross-domain MDA MnS consumers.
- Core Network (CN) Domain Interaction:
- The cross-domain MDA MnS producer interacts with CN domain MDA MnS producers to consume analytics results generated by NWDAF, MnS provided by CN domain management, or other sources like element and subnetwork management functions.
- This interaction ensures that insights from the CN domain are integrated into cross-domain analytics to enhance system-wide management capabilities.
- Radio Access Network (RAN) Domain Interaction:
- Similarly, the cross-domain MDA MnS producer interacts with RAN domain MDA MnS producers to consume MnS or analytics results from the RAN domain.
- The insights derived from RAN-specific data are used to produce broader cross-domain analytics services that can support 3GPP-wide use cases.
- Unified Analytics for Complex Use Cases:
- By consuming and synthesizing data from both CN and RAN domains, cross-domain MDA enables analytics-driven decision-making that spans multiple network layers and domains.
- This approach ensures that network operators have access to integrated insights, facilitating end-to-end network optimization, fault detection, and predictive analytics.
This is an example of cross-domain Management Data Analytics (MDA) within the 3GPP framework, demonstrating the interaction between different domains and the flow of analytics information. It highlights how cross-domain MDA facilitates the integration and collaboration between analytics producers and consumers across the Core Network (CN) and 3GPP cross-domain management levels.
This example shows the modularity and interoperability of the 3GPP MDA framework. It showcases how the cross-domain MDA aggregates and synthesizes data from domain-specific analytics systems, such as the Core Network, to produce unified insights. These insights are crucial for enabling intelligent, analytics-driven management across diverse network layers and domains.
< TS 28.104 - Figure 5.3-1 >
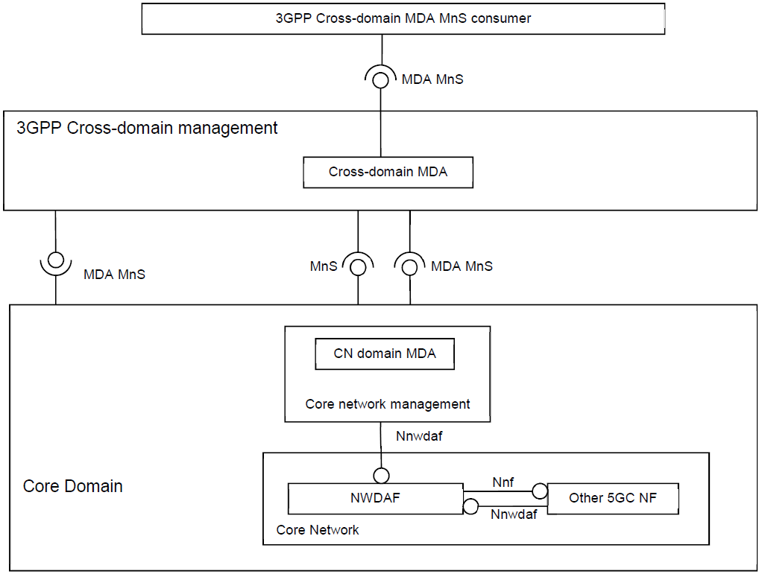
Followings are breakdown and description about the architecture shown above :
- 3GPP Cross-Domain Management:
- At the top of the hierarchy, the 3GPP cross-domain MDA is responsible for synthesizing and analyzing data from multiple domains, including the Core Network and other network components.
- This cross-domain management acts as an intermediary, aggregating data from domain-specific MDAs and producing actionable Management and Service (MnS) analytics for 3GPP-wide use cases.
- Cross-Domain MDA:
- The central "Cross-Domain MDA" within the 3GPP cross-domain management layer consumes analytics and management services (MnS) from the Core Domain.
- It processes this data to generate cross-domain insights and services (MDA MnS), which are subsequently consumed by the 3GPP cross-domain MDA MnS consumer.
- Core Domain:
- Within the Core Domain, the Core Network MDA (CN Domain MDA) plays the role of a domain-specific analytics producer.
- It interacts with multiple components of the Core Network, including NWDAF (Network Data Analytics Function) and other 5G Core Network Functions (5GC NFs), to collect and analyze data.
- NWDAF provides specialized analytics results based on data derived from the core network, which are consumed by the CN domain MDA.
- Flow of Data and MnS:
- Data flows from the NWDAF and other 5GC NFs to the CN domain MDA. This data includes analytics results, key performance indicators (KPIs), and other operational metrics.
- The CN domain MDA then provides MnS to the Cross-Domain MDA, which integrates this data with other sources and generates cross-domain analytics outputs.
- The cross-domain analytics outputs are delivered to the 3GPP cross-domain MDA MnS consumer for decision-making and optimization.
- End-to-End Integration:
- This example demonstrates how the MDA framework ensures seamless collaboration between domain-specific analytics (e.g., CN domain) and overarching cross-domain management.
- By integrating insights across domains, the cross-domain MDA enables holistic network management, addressing complex scenarios such as resource optimization, fault detection, and service assurance across 5G networks.
This is an example of coordination between the Cross-Domain MDA and the RAN Domain MDA within the 3GPP framework. It demonstrates how the cross-domain management layer interacts with the Radio Access Network (RAN) domain to facilitate analytics-driven network management and optimization.
This structure exemplifies a modular and hierarchical approach to network management analytics. The RAN domain MDA focuses on domain-specific insights, while the cross-domain MDA synthesizes these insights with data from other domains to provide unified, actionable analytics. This coordination ensures efficient and optimized management of both the RAN and the broader 5G network.
< TS 28.104 - Figure 5.3-2 Example of coordination cross-domain MDA and RAN domain MDA>
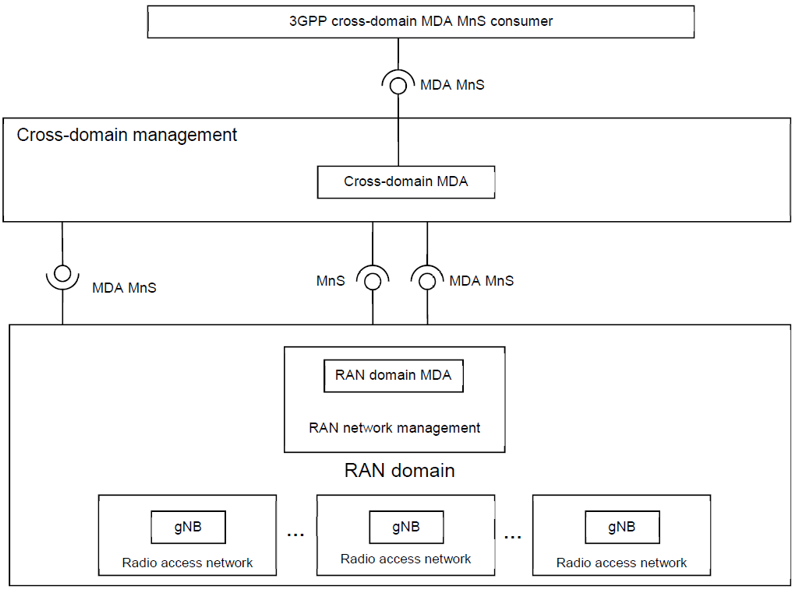
Followings are the breakdown and description of the structure
- 3GPP Cross-Domain MDA MnS Consumer:
- At the top of the structure is the MDA MnS Consumer, which consumes the analytics services (MDA MnS) generated by the cross-domain MDA.
- These consumers could be external entities or systems responsible for managing and optimizing the overall network based on the insights provided.
- Cross-Domain Management:
- The Cross-Domain MDA acts as the central analytics producer in the cross-domain management layer.
- It consumes data from the RAN domain MDA and other sources, synthesizes insights, and provides MDA MnS to the 3GPP cross-domain MDA MnS consumer.
- RAN Domain MDA:
- The RAN Domain MDA focuses on analytics specific to the RAN, such as data related to gNBs (next-generation NodeBs) and their operations.
- It interacts with the RAN network management system to consume and produce MnS that is relevant to the RAN domain.
- This MDA instance processes analytics at the domain level, addressing issues like traffic balancing, resource optimization, and fault management within the RAN.
- Radio Access Network (RAN) Domain:
- The RAN domain consists of multiple gNBs, which are the base stations of the 5G network. These gNBs generate data that is consumed by the RAN domain MDA for analytics.
- This data includes network traffic statistics, resource usage patterns, and fault logs, which are critical for maintaining efficient RAN operations.
- Data and MnS Flow:
- From RAN Domain to RAN Domain MDA: gNBs provide data to the RAN domain MDA, which processes this information to generate MnS.
- From RAN Domain MDA to Cross-Domain MDA: The RAN domain MDA shares its analytics outputs (MnS) with the cross-domain MDA for further synthesis.
- From Cross-Domain MDA to Consumers: The cross-domain MDA produces higher-level MDA MnS, integrating RAN-specific analytics with data from other domains, and delivers this to the 3GPP cross-domain MDA MnS consumer.
MDA use cases covers almost entire aspects and functions of core network and RANs. There are extensive list and details described in TR 28.809. In this note, I will put down short descriptions of each use cases described in the TR. Check out TR 28.809 if you are interested in further details of a specific use case.
Slice Coverage Optimization
This use case focuses on ensuring that network slices provide optimal geographic and service coverage. It involves mapping slice-specific requirements, such as capacity and quality, to the available RAN resources. The process includes analyzing mobility patterns, user density, and access node capabilities to dynamically adjust the Tracking Area (TA) and Registration Area (RA) configurations. This optimization reduces resource wastage while maintaining service-level agreements (SLAs) for coverage and quality.
- Description: This use case involves optimizing the geographical coverage of network slices to meet Service Level Agreements (SLAs) efficiently. It focuses on mapping the desired slice coverage to actual radio coverage during slice instantiation and runtime.
- Key Points:
- Includes considerations for slice-aware statistics, mobility patterns, and access node capabilities.
- Optimizes Tracking Area (TA) and Registration Area (RA) configurations to reduce resource usage while fulfilling coverage requirements.
RAN User Plane Congestion Analysis
This use case identifies and addresses congestion in the RAN's user plane, where data traffic is managed. Congestion can result in degraded user experience and QoS violations. The analysis focuses on pinpointing traffic bottlenecks caused by high resource usage or uneven load distribution. Insights from this analysis help in implementing measures like traffic prioritization, resource reallocation, or load balancing to alleviate congestion.
- Description: This analyzes congestion in the RAN user plane to identify bottlenecks and their root causes.
- Key Points:
- Aims to ensure Quality of Service (QoS) by mitigating congestion impacts.
- Provides insights for resource reallocation and prioritization strategies
Resource Utilization Analytics
This use case involves detailed monitoring and analysis of how network resources, such as spectrum and hardware, are utilized. By identifying overutilized and underutilized resources, the system can suggest adjustments to improve efficiency. For example, underutilized resources in one area might be reallocated to more demanding regions, ensuring balanced network operations.
- Description: Focused on analyzing how resources (e.g., spectrum, hardware) are utilized within the network.
- Key Points:
- Identifies underutilized or overutilized resources.
- Suggests optimization actions to balance the load and improve efficiency
Cross-Slice Resource Optimization
This use case ensures fair and efficient distribution of resources across multiple network slices. The system evaluates resource allocation for each slice to avoid inter-slice conflicts and underutilization. By harmonizing resource usage across slices, it helps maintain overall network performance while meeting the requirements of individual slices.
- Description: This ensures that resources are optimally distributed across multiple network slices.
- Key Points:
- Balances resource allocation between slices to maintain overall network performance.
- Addresses inter-slice dependencies and conflicts
NAS Level Congestion Control Optimization
At the Non-Access Stratum (NAS) level, this use case addresses signaling congestion caused by an overload of control messages. By analyzing signaling traffic patterns and processing delays, the system identifies congestion points. Optimization strategies may include prioritizing critical signaling messages and throttling non-urgent requests.
- Description: Addresses congestion issues at the Non-Access Stratum (NAS) level, focusing on signaling control.
- Key Points:
- Optimizes signaling load and reduces delays in NAS message processing
E2E Latency Analysis
This use case examines latency across the entire service path, from the user device to the application server. By identifying latency contributors in the RAN, core, and transport networks, the system provides insights for reducing delays. This is especially critical for URLLC services that require extremely low latency.
- Description: This use case focuses on identifying and mitigating latency issues across the end-to-end (E2E) service path.
- Key Points:
- Analyzes latency contributors from RAN, Core Network (CN), and Transport Network (TN).
- Provides actionable insights to ensure compliance with Ultra-Reliable Low-Latency Communication (URLLC) requirement
Network Slice Load Analysis
This use case evaluates the load distribution within network slices. It identifies slices that are overloaded or underutilized, enabling proactive resource adjustments. For example, overloaded slices may receive additional resources, while underutilized ones might release theirs, ensuring better resource utilization.
- Description: Analyzes the load distribution within network slices to prevent overload or underutilization.
- Key Points:
- Identifies load variations across slices and recommends resource adjustments.
- Supports SLA assurance through load distribution analysis
Service Experience Analysis
This use case focuses on analyzing the quality of service experienced by end users. Metrics such as latency, throughput, and reliability are monitored to identify performance issues. The analysis helps operators understand user satisfaction levels and take corrective actions to improve service quality.
- Description: Evaluates the quality of service experienced by end users.
- Key Points:
- Identifies issues affecting user experience, including latency, throughput, and connectivity.
- Recommends improvements for better service delivery
Network Slice Throughput Analysis
This use case examines the data throughput performance of individual network slices. It highlights any deviations from expected throughput levels, helping operators identify and address throughput bottlenecks to ensure slice SLAs are met.
- Description: Examines throughput performance for each network slice.
- Key Points:
- Identifies throughput bottlenecks and suggests improvements.
- Ensures slices meet their throughput SLAs
Uplink/Downlink Throughput per UE in Network Slice Analysis
This use case focuses on monitoring and analyzing the uplink and downlink throughput of individual user equipment (UE) within a specific slice. It provides granular insights into the performance of each UE, enabling targeted optimizations for better user experience.
- Description: Monitors throughput at the UE level within a specific slice.
- Key Points:
- Provides detailed uplink and downlink performance metrics.
- Helps in optimizing slice-specific configurations
KPI Anomaly Analysis
This use case detects anomalies in Key Performance Indicators (KPIs) such as latency, packet loss, and throughput. By identifying outliers and irregular patterns, the system can pinpoint potential issues before they escalate into larger problems, ensuring network stability and reliability.
- Description: Identifies anomalies in Key Performance Indicators (KPIs) that may indicate network issues.
- Key Points:
- Uses analytics to pinpoint root causes.
- Helps in maintaining network reliability and performance
Jitter Analysis
Jitter refers to variations in packet delay, which can disrupt real-time applications like voice and video. This use case analyzes jitter patterns, identifies root causes, and suggests mitigation strategies to stabilize the network and enhance performance for time-sensitive applications.
- Description: Focuses on analyzing and mitigating packet delay variations (jitter) that affect real-time services.
- Key Points:
- Supports performance tuning for services like voice and video.
- Provides insights into network stability
Network Slice Traffic Projection
This use case uses historical and real-time traffic data to forecast future traffic trends for network slices. These projections help operators plan resource allocations proactively, ensuring that slices are adequately prepared for expected traffic surges or seasonal variations.
- Description: Predicts traffic trends for network slices to facilitate resource planning.
- Key Points:
- Helps in proactive resource allocation based on traffic forecasts.
- Involves detailed modeling of traffic load pattern
Fault Management Related Issues
Fault management focuses on identifying and resolving issues in the network. This includes real-time fault detection, historical fault pattern analysis, and predictive fault analytics to prevent failures before they occur. Examples include detecting alarm incidents, predicting equipment malfunctions, and analyzing alarm inaccuracies.
- Description: Involves analytics for identifying and resolving network faults proactively.
- Key Points:
- Includes failure prediction, alarm incident analysis, and alarm malfunction analytics
Handover Optimization
This use case improves the handover process as UEs move between cells. By analyzing trajectory patterns, resource availability, and network conditions, the system recommends optimal handover parameters to ensure seamless connectivity and minimal disruption during mobility.
- Description: Focuses on improving the handover process to ensure seamless mobility.
- Key Points:
- Analyzes UE trajectory, load conditions, and target cell resources.
- Recommends optimal handover parameters
Energy Efficiency Related Issues
Energy efficiency aims to reduce power consumption across the network. By identifying high-energy-consuming components and optimizing their operation, this use case helps operators save energy while maintaining network performance. Examples include turning off idle resources or adjusting transmission power levels during low traffic periods.
- Description: Addresses energy consumption challenges in the network.
- Key Points:
- Identifies high-energy-consuming components.
- Suggests energy-saving configurations and operational changes
RAN Node Software Upgrade
This use case optimizes the software upgrade process for RAN nodes, minimizing service disruption. By analyzing upgrade windows and network traffic patterns, the system schedules upgrades during low-traffic periods and ensures a smooth transition with minimal impact on services.
- Description: Optimizes software upgrade processes to minimize service disruption.
- Key Points:
- Provides analytics for identifying optimal upgrade windows.
- Reduces operational costs and service impact
SON Conflict Prevention and Resolution
Self-Organizing Networks (SON) functions can sometimes conflict, leading to inefficiencies or performance degradation. This use case analyzes and resolves such conflicts, ensuring that SON features like load balancing and mobility management work harmoniously to enhance network efficiency.
- Description: Deals with conflicts in Self-Organizing Network (SON) operations.
- Key Points:
- Resolves overlapping or conflicting SON functions.
- Improves network efficiency and stability
Security Risk Assessment
This use case evaluates the network's vulnerability to security threats. By analyzing potential risks and existing safeguards, the system provides recommendations to strengthen network security, ensuring robust protection against attacks and data breaches.
- Description: Evaluates and mitigates security risks in the network.
- Key Points:
- Identifies vulnerabilities and threats.
- Recommends security measures to safeguard network operation
Reference
YouTubes
|
|