Our Brain's Learning Limits
Have you ever wondered why some skills feel easy to pick up, while others seem very hard no matter how much you try? The human brain is an amazing tool, able to learn languages, play music, or improve at sports like basketball. But even with all its power, it’s not a limitless machine that can adapt to everything. Deep inside its connections, there are limits—rules built into it that decide what we can and cannot do. Scientists have recently found new ideas about these secret boundaries, showing
that the way our brain cells are linked might explain why some things feel simple and others stay impossible. In this note, we’ll explore an exciting discovery from neuroscience that reveals how our brain’s "wiring" shapes what we can really learn.
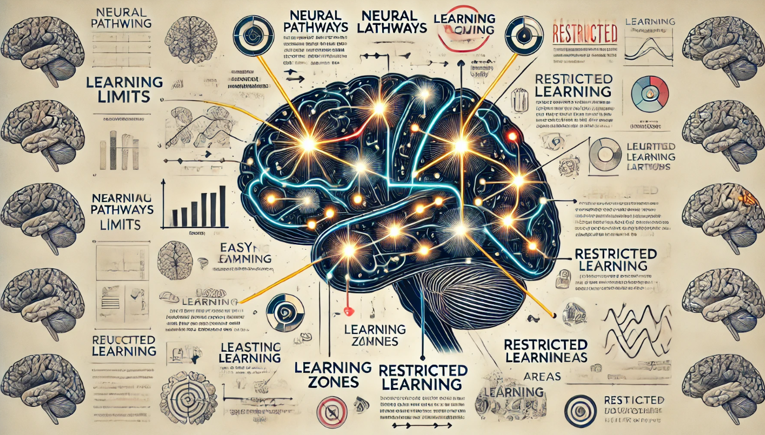
Research shows a new way of thinking about how we learn. Just as a river is shaped by the terrain, our thoughts, movements, and abilities are shaped by the physical structure of our brains. Understanding these constraints may help us develop more effective learning strategies, training techniques, and even new approaches to rehabilitation for individuals with neurological injuries. While our brains are remarkably adaptable, they are not limitless—and recognizing these natural boundaries
could be the key to unlocking our full potential.
Why Can’t We Learn Everything?
Have you ever found yourself struggling to master a new skill, no matter how much effort you put in? While practice and dedication are essential for learning, research suggests that our brains have built-in constraints that determine what we can and cannot master. These constraints aren’t just about motivation or repetition; they are deeply rooted in the way our neurons are wired and how they process information. Just like rivers following natural paths shaped by the landscape, neural activity
follows preferred pathways that make some learning easier and some nearly impossible.
Recent research, such as the study "Dynamical Constraints on Neural Population Activity" has provided insights into why mastering certain skills can be difficult despite dedicated practice. Key findings include:
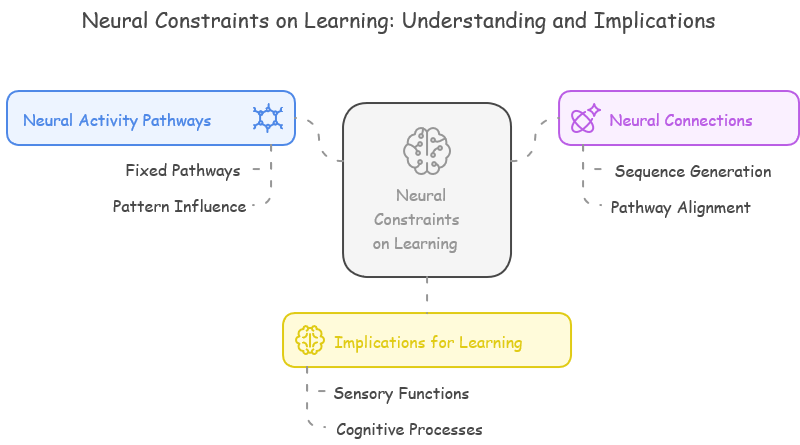
- Neural Activity Follows Fixed Pathways: The brain’s electrical signals do not move randomly; they follow specific patterns dictated by the brain’s physical structure. These patterns influence how we process information and determine which learning sequences are naturally easier or harder to acquire.
- Pre-existing Neural Connections Shape Learning: The way neurons are connected determines how easily certain neural activity sequences can be generated. If a new skill requires a sequence that does not align with these existing pathways, learning becomes significantly more challenging.
- Implications for Learning and Behavior: These constraints affect not just sensory and motor functions but also cognitive processes. Understanding these built-in limitations can help refine learning strategies, making skill development more effective by working with the brain’s natural dynamics rather than against them.
Examples of Hard-to-Learn things
Here are some examples of what our brain struggles to learn due to its inherent neural constraints. These findings highlight that while the brain is highly adaptable, its learning ability is shaped by predefined neural structures and preferred activity patterns, making some skills much harder - if not impossible - to learn.
- Reversing Complex Motor Sequences : The brain cannot easily execute learned motor sequences in reverse order. For example, if you learn to type a sentence fluently, trying to type it backward (letter by letter) is extremely difficult because the neural pathways are optimized for the forward sequence.
- Generating Arbitrary Neural Patterns : When using a Brain-Computer Interface (BCI), participants were able to generate some new neural activity patterns but failed to create arbitrary or unnatural ones. This suggests that the brain is constrained by existing pathways and cannot generate completely novel patterns at will.
- Mirror-Reversing Movements : If you train one hand to perform a complex sequence (like playing a piano scale), it is difficult to directly mirror that movement with the other hand, even though both hands are capable of similar actions. The neural circuits controlling each hand operate differently, making mirrored execution unnatural.
- Replaying Neural Activity in Reverse : Even with feedback and motivation, subjects in the study could not perfectly reverse the neural trajectories associated with a learned movement. This suggests that once a sequence is encoded in the brain’s activity, reversing it is not simply a matter of playing it backward.
- Altering the Timing of Neural Activation : The brain follows preferred time scales for processing and movement execution. For example, artificially speeding up or slowing down a movement beyond natural limits leads to a loss of coordination, showing that the brain struggles to learn actions that disrupt its built-in temporal dynamics.
- Learning Completely Unrelated Motor Patterns : Tasks that require completely new and unnatural coordination (e.g., moving each finger in a different rhythmic pattern at the same time) are extremely difficult because they conflict with the brain’s existing motor pathways.
The Challenge of Studying Neural Constraints
Studying these constraints scientifically is a complex challenge. When you perform an action, neurons across your brain fire in intricate sequences. But how do we determine which neural patterns are easy to generate and which are difficult? One way to approach this question is through systematic testing: trying different movement sequences and analyzing which ones the brain struggles to learn. However, traditional behavioral experiments don’t allow us to directly control or observe neural activity,
making it difficult to pinpoint the exact limitations of our brain's learning capacity.
Followings are scientific challenges for investigation and how to overcome the challenges:
- Complexity of Neural Activity Patterns: When performing actions, neurons fire in intricate sequences, making it difficult to discern which patterns are easily generated and which are not.
- Limitations of Traditional Behavioral Experiments: Conventional methods don't allow direct control or observation of neural activity, hindering precise identification of the brain's learning limitations.
- Innovations in Research Methodologies: To overcome these challenges, researchers have employed brain-computer interfaces (BCIs) to directly link neural activity with external devices. This approach enables the study of how the brain adapts to new neural sequences and identifies patterns that are difficult to produce.
- Findings on Neural Constraints: Studies using BCIs have revealed that subjects struggle to generate neural activity sequences that contradict the brain's intrinsic temporal patterns, highlighting fundamental limitations in our learning processes.
Following figure consists of two panels labeled (a) and (b), illustrating how neural population activity is mapped to movement through a Brain-Computer Interface (BCI) system. This figure demonstrates that the brain does not generate movement arbitrarily; instead, it follows specific neural pathways that constrain how actions are performed. The experiment likely investigates whether certain movement sequences are more difficult to learn due to the brain's natural wiring and preferred neural
activity flow. The findings reinforce the idea that neural constraints limit the flexibility of motor learning, which has implications for neuroprosthetics, rehabilitation, and skill acquisition.
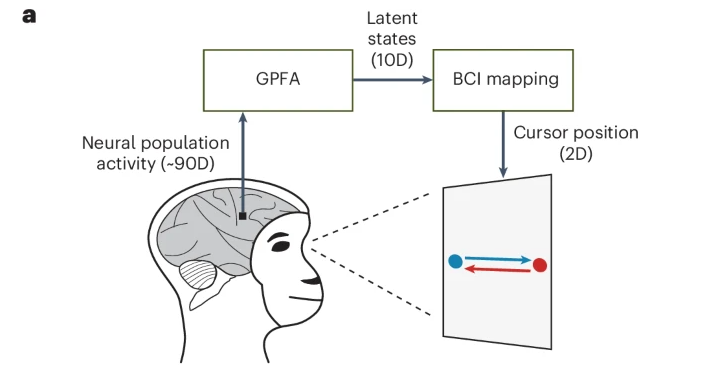
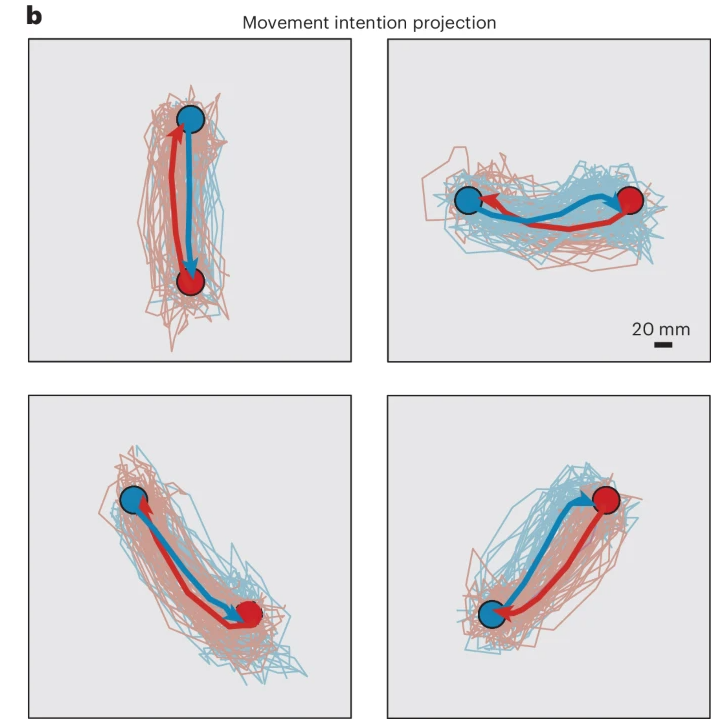
Image Source : Dynamical constraints on neural population activity
- Panel (a): Neural Population Activity to Cursor Movement
- This diagram shows how neural signals from the brain are transformed into movement control via a BCI system.
- Neural population activity (~90D): The brain generates complex, high-dimensional neural activity (~90 dimensions) as a person intends to move.
- GPFA (Gaussian Process Factor Analysis): This technique is used to reduce the dimensionality of neural signals, extracting latent states (simplified neural representations in 10D).
- BCI Mapping: The extracted 10D latent states are further translated into a 2D cursor position on a screen, allowing the user to control movement using only brain activity.
- Visual feedback: The subject observes the cursor moving between targets (blue and red circles), helping to reinforce the learning process.
- Panel (b): Movement Intention Projection
- This section visualizes how neural activity translates into cursor movements under different conditions.
- Each subplot represents a different movement trajectory between two points (red and blue circles).
- The colored lines (red and blue) indicate movement in different directions.
- The overlapping trajectories (faint background traces) show multiple trials, highlighting consistency or variability in the movement paths.
- Key observation: The movement paths follow specific patterns, suggesting that neural activity constrains how movements are planned and executed.
- Top-Left : Straight Movement Path
- The movement trajectory is mostly linear and follows a well-defined path between the two target points.
- The red and blue lines (indicating movement directions) overlap closely, meaning the brain is efficiently generating similar patterns for both forward and backward movements.
- This suggests that the movement direction aligns well with the brain’s preferred neural activity pathways.
- Top-Right : Curved Movement Path
- Here, the movement path deviates from a straight line and follows a noticeable curved trajectory.
- The background traces show significant variability, meaning the subject struggled to maintain a consistent trajectory across trials.
- This could indicate that the neural activity required for this movement does not align naturally with the brain’s internal structure, making it harder to execute.
- Bottom-Left : Another Straight Movement Path with Minor Variability
- Similar to the top-left image, this trajectory is mostly straight and consistent, but with slightly more variation in the background traces.
- The movement appears smoother and more repeatable, suggesting that this movement sequence aligns well with the brain’s natural motor planning capabilities.
- The presence of slight variation might indicate subtle challenges in maintaining perfect consistency.
- Bottom-Right : Asymmetrical Curved Movement
- This movement is highly curved, and the red and blue paths do not overlap symmetrically.
- The deviation from a straight trajectory suggests neural constraints are preventing the subject from generating an ideal mirrored movement.
- The brain’s wiring may favor one direction over the other, causing difficulties in reversing the movement naturally.
Reference
YouTube/Other Video
|
|