Dish Neuron / In Vitro Neuron
In-vitro neurons are brain cells grown in a lab, not inside a body. Scientists do this to learn more about how these cells work on their own. This method lets them see how neurons communicate, respond to different situations, and change over time without the complexity of the whole brain. This research helps us understand how the brain functions, what goes wrong in brain diseases, and how to find new treatments. It's also used in creating new technologies that combine living cells with electronic
devices.
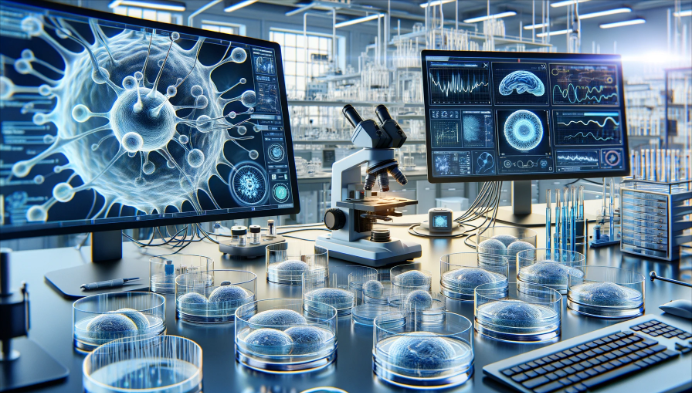
Followings are some of the bullets about this futuristic / science-fiction like technology
The use of in-vitro neurons, or brain cells grown in a lab, has many important uses. It helps scientists learn more about how our brain works and find new ways to treat brain diseases. This technology is key in discovering new medicines by testing how different drugs affect these cells. Also, it plays a big role in connecting the human brain with computers, leading to new inventions that can help people communicate or think better. This research is not just about treating illnesses but also
about making new discoveries in technology and understanding more about how we think and feel.
- Neuroscience Research: It provides a detailed understanding of how neurons work, communicate, and develop. This is crucial for unraveling the complexities of the brain and nervous system.
- Drug Discovery and Testing: In-vitro neurons allow for the testing of new drugs and treatments for neurological disorders in a controlled environment, speeding up the development of effective therapies.
- Disease Modeling: Researchers can model neurological diseases using in-vitro neurons, helping them study disease mechanisms and identify potential therapeutic targets.
- Regenerative Medicine: This technology contributes to the development of stem cell therapies aimed at repairing or replacing damaged neurons in conditions like spinal cord injuries or neurodegenerative diseases.
- Brain-Computer Interfaces (BCIs): By integrating in-vitro neurons with electronic devices, scientists are paving the way for advanced BCIs that could help restore lost sensory or motor functions.
- Educational Tools: In-vitro neuron cultures serve as valuable educational tools for students and researchers learning about neurobiology and the functioning of the nervous system.
- Synthetic Biological Intelligence: As seen in the DishBrain system, in-vitro neurons can be used to create synthetic biological intelligence, opening new avenues in computing and artificial intelligence research.
Growing brain cells in a lab, using in-vitro neuron technology, is a big step forward in science. However, it's important to look at the problems and questions it brings up. Here, we talk about the difficulties, like how complex and expensive the process is, and the ethical concerns about using human cells. We also discuss why what works in the lab might not always work in real life. Understanding these issues helps us see the full picture of this technology and what needs to be done to make
it better and more useful for everyone.
Here comes with its set of drawbacks and challenges:
- Complexity and Cost: Culturing neurons in-vitro requires sophisticated equipment, controlled environments, and significant expertise, making the process complex and costly.
- Limited Context: While in-vitro models allow for controlled study of neuronal behavior, they lack the full complexity of living organisms. This absence of a complete biological context can limit the understanding of how neurons operate within the natural environment of a living brain.
- Scalability: Scaling up in-vitro neuron cultures for large-scale applications or treatments poses significant challenges, including maintaining cell health and function over time.
- Translation to Clinical Use: There is always a gap between laboratory findings and their application in real-world clinical settings. Results observed in cultured neurons might not always translate directly to effective treatments in humans due to the complexity of the human body and brain.
- Longevity and Stability: Maintaining the viability and functionality of in-vitro neurons over extended periods is challenging, which can limit long-term studies or applications.
- Artificial Environment: The artificial environment in which in-vitro neurons are grown may not perfectly mimic the conditions within a living brain, potentially affecting the cells' behavior and responses in ways that are not fully representative of natural neuronal function.
- Ethical Concerns: The use of human neuronal cells raises ethical questions, especially when it comes to sourcing these cells, consent, and the potential for creating consciousness or pain perception in vitro.
This shows a dish of cultured neurons (in-vitro neurons) that are part of a biohybrid system interfacing with a computer simulation of the game "Pong." The diagram illustrates how the neurons receive sensory input and produce motor outputs to interact with the game, with prediction errors and sensory perceptions being critical components of the system. The setup aims to study how these neurons can adapt and learn within this simulated environment.
How the test works ?
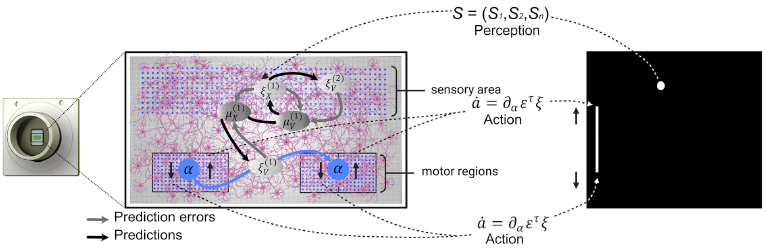
Image Source : In vitro neurons learn and exhibit sentience when embodied in a simulated game-world )
NOTE : Check out this video : Lab-Grown "Mini-Brain" Learns Pong - Is This Biological Neural Network "Sentient"? if you have difficulties understanding how this works just by reading.
This is what and how this setup works. (NOTE : most of the details here came from In vitro neurons learn and exhibit sentience when embodied in a simulated game-world )
- Sensory Area and Electrodes: The neurons were grown on a high-density multielectrode array with a predefined sensory area made up of 8 electrodes. These electrodes provided the neurons with information about the game.
- Motor Regions and Paddle Movement: Two separate motor regions were identified, which corresponded to the movement of the paddle in the Pong game. One region controlled the paddle's upward movement and the other controlled the downward movement.
- Playing the Game: The electrophysiological activity (the neurons' activity) was used in real-time to control the paddle. If the neurons' activity led to the paddle hitting the ball, the game would continue.
- Feedback Through Stimulation:
- If the neurons failed to intercept the ball with the paddle, an unpredictable stimulus was applied: a 150mV voltage at 5Hz for 4 seconds. This was meant to simulate a form of "punishment" for missing the ball.
- If the paddle successfully hit the ball, a predictable stimulus was given across all electrodes simultaneously at 100Hz for 10ms. This served as a "reward" for successfully hitting the ball.
- NOTE : how neuron can recognize the unpredictable stimulus as punishment and predictable stimulus as reward when they don't have any high level cognitive capability ?
- Unpredictable Stimulus as Punishment: An unpredictable stimulus is considered a punishment because it disrupts the pattern of stimuli that the neurons are starting to understand or predict. It serves as a negative feedback signal, indicating that the previous action did not have the desired outcome. This unpredictability is key because it signals to the neurons that their current behavior is not achieving the intended effect, prompting them to adjust their firing patterns.
- Predictable Stimulus as Reward: A predictable stimulus acts as a reward because it confirms the neurons' predictions about the environment. When neurons successfully predict the sensory consequences of their actions, the predictable nature of the feedback reinforces that behavior. In the case of DishBrain, successfully hitting the ball with the paddle led to a brief, predictable electrical pulse, which would be 'perceived' by the neurons as a positive outcome.
- NOTE : How we can implement 'unpredictability' ?
- Based on common practices in similar experiments, unpredictability could be implemented in several ways:
- Random Timing: The stimulus could be delivered at random intervals rather than in a consistent pattern.
- Random Intensity: The strength of the electrical signals could vary unpredictably in amplitude.
- Random Location: The electrical pulses could be delivered to random electrodes rather than the same electrode each time.
- Random Frequency: The frequency of the pulses could change, so the neurons cannot anticipate when the next pulse will occur.
- Learning and Adaptation: The idea was that the neurons would self-organize and adapt their firing patterns to better control the paddle and hit the ball more consistently, thus learning to play Pong through a process of trial and error, similar to how a human or animal might learn through consequences.
- Testing and Results: The experiment included tests with different configurations of the motor regions to ensure that there was no bias in the neurons' response due to the setup. The neurons demonstrated the ability to hit the ball more consistently over time, indicating a form of learning and adaptation.
- Self-Organization: The fixed layout of the motor regions meant that the neurons had to develop distinct firing patterns on their own to control the paddle, raising questions about the extent of self-organization that could occur
Why this test ?
The DishBrain experiment sought to bridge the gap between biological intelligence and computational models, offering a new way to look at how neurons function individually and as part of larger networks.
This experiment was multi-faceted as follows:
- To Demonstrate Adaptive Behavior In-Vitro: The experiment aimed to show that biological neural networks (BNNs), when placed in a simulated environment, could adapt their behavior in real-time in response to stimuli. This is a fundamental aspect of learning that is well established in living organisms (in vivo), but this was the first time it was established in a lab setting (in vitro) for goal-directed behavior.
- Investigation of Neuronal Computation: The system was used to investigate the fundamentals of how biological neurons process information and compute responses to stimuli. This could help understand how neurons carry out complex tasks such as spatial and non-spatial problem-solving, which had been previously modeled in silico (using computer simulations).
- Technical Advancement: The experiment represented a significant technical step forward by creating a closed-loop environment for BNNs. A closed-loop system is one in which the outputs (or responses) of the system are fed back into it as inputs, creating a self-regulatory system. This setup allows for the dynamic interaction between the neural activity and the environment, which is essential for adaptive learning.
- Data Generation on Neuronal Processing: The DishBrain platform allowed researchers to test different conditions, such as cell types, drug effects, and feedback scenarios, to gather new data on how cells process information and respond to changes in their environment. This data could provide insights that were not previously attainable due to the limitations of in silico models or the inability to observe such phenomena directly in vivo
Artificial intelligence has come a long way in recent years, but a new technology called Synthetic Biological Intelligence (SBI) is about to turn everything on its head. Imagine a computer not just made of silicon, but also living human brain cells—actual neurons grown in a lab, learning and adapting in real time. It might sound like science fiction, but it’s real, it’s called the CL1, and you can already buy it.
Again this isn’t a plot twist from a sci-fi blockbuster—it’s real, it’s here, and it’s available for purchase. Scientists at Cortical Labs have unveiled the CL1, a groundbreaking "biological computer" that fuses lab-grown neurons with silicon hardware to create the SBI. Launched on March 2, 2025, in Barcelona, this tech promises to outpace traditional AI chips in speed, efficiency, and adaptability. Let’s dive into what makes this innovation so wild—and why it might just change everything.
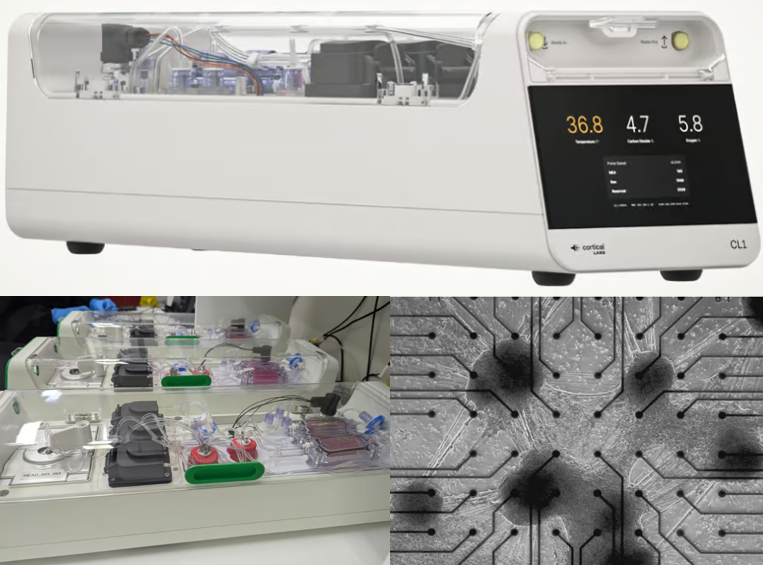
What Is the CL1, Exactly?
At its core, the CL1 is a hybrid of biology and technology. It starts with human neurons—grown in a lab from induced pluripotent stem cells (iPSCs), which are essentially adult cells reprogrammed to act like embryonic stem cells. These neurons are placed onto a silicon chip equipped with 59 electrodes arranged in a grid. This setup acts like a playground where the neurons can grow, connect, and form a living neural network. Cortical Labs describes it as a "body in a box," complete
with a life-support system—filtration pumps, temperature controls, and gas mixing—to keep these cells thriving.
Unlike traditional computers that rely solely on silicon and code, the CL1 integrates this organic network with software that communicates with the neurons in real time. It sends electrical signals to the cells and reads their responses, creating a feedback loop. The neurons, which naturally seek energy-efficient ways to process signals, can be "rewarded" with predictable inputs when they perform a task correctly or "punished" with chaotic signals when they don’t. This isn’t
just theory—it’s been proven. Back in 2022, Cortical Labs’ earlier project, DishBrain, taught a similar neural network to play Pong using this reward-and-punishment method, making headlines and laying the groundwork for the CL1.
- Living Neurons: Cultured from induced pluripotent stem cells (iPSCs).
- Planar Electrode Arrays: A grid of 59 electrodes that both read the electrical signals from the neurons and write (stimulate) signals back to them.
- “Body in a Box” Environment: Life-support systems maintain temperature, gas mix, and nutrient supply so the neurons stay healthy.
- Built-In Software & Touchscreen: No separate computer required; the CL1 is entirely self-contained, with a display to monitor and control experiments in real time.
- Price Tag: About $35,000 for one standalone unit.
- Power Usage: A CL1 rack containing 30 units runs on just under 1 kilowatt—about the same as a small microwave. A single CL1 unit is even more efficient.
How It Works
The CL1 from Cortical Labs represents a stunning fusion of biology and technology, powered by a living network of lab-grown human brain cells. It begins with induced pluripotent stem cells (iPSCs), which are coaxed into becoming neurons and then cultivated on an electrode grid, forming a responsive, organic system. This network interacts with the digital world through electrical stimulation: software sends signals to the neurons—say, indicating a Pong paddle’s position—and the neurons fire
back, driving actions like moving the paddle. The system learns through a feedback loop, rewarding "correct" responses with predictable patterns and discouraging errors with chaotic ones, mirroring how traditional neural networks train, but with real, living cells. Underpinning this is the "Minimal Viable Brain" concept, where Cortical Labs pinpoints the smallest, most efficient neuronal configuration needed for learning, enhancing the CL1’s stability and programmability. This isn’t just a
computer—it’s a new paradigm of intelligence.
- Lab-Grown Neurons
- The human brain cells used in CL1 start out as iPSCs, which can differentiate into virtually any cell type. These neurons then grow on top of an electrode grid, forming a living, responsive network.
- Electrical Stimulation & Feedback
- Input: An electrical signal tells the neurons what’s happening in a virtual environment (for instance, the position of a Pong paddle).
- Output: The neurons fire back, prompting the software to move the paddle or otherwise respond.
- Rewards & Punishments: Predictable electrical patterns reinforce “correct” moves, while chaotic signals represent “mistakes.” Over time, the neurons learn to optimize their responses, much like training a neural network in software—but with real brain cells.
- Minimal Viable Brain Concept
- Cortical Labs’ research identifies the smallest set of neurons (and neuron types) necessary for robust learning. This streamlines the technology, making each CL1 unit more stable and easier to program.
Real-World Applications
The CL1’s SBI brings many exciting real-world possibilities by using living human neurons in a way that traditional AI cannot do. This new method takes advantage of the special abilities of biological systems, giving a lively and natural base that is different from the usual hard, silicon-based AI setups. By mixing these living cells into a computing system, the CL1 opens doors to big changes in many areas, using the natural speed and flexibility of human neurons to solve tough problems that normal digital ways struggle with. It combines biology and technology not just to copy intelligence but to build on what life already offers, promising to change how we work on new ideas in science, medicine, and more. Followings are some of the possible applications that we can think of as of now :
- Drug Discovery & Disease Modeling : Because these neurons are biologically human, researchers can study neurological conditions in a living network. This could dramatically improve how new drugs are tested, especially for complex brain disorders like Alzheimer’s, epilepsy, and Parkinson’s. Rather than relying on 2D petri dish models or animal studies, scientists can use a more realistic human-derived neural substrate to test drug efficacy and toxicity.
- Personalized Medicine : Since neurons can be grown from a patient’s own cells, a doctor could foreseeably create a personalized neural culture to see how that individual’s brain cells respond to a medication—lowering the risk of adverse reactions or treatment failure.
- Robotics & Automation : Today’s AI-run robots rely on massive datasets and algorithmic training. SBI-based robots could learn more flexibly, adapting in real-time based on dynamic feedback loops. The “living neural network” might make robotics more energy-efficient and better at handling novel, unpredictable situations.
- Next-Gen AI Systems : Large language models (like ChatGPT) run on power-hungry data centers filled with GPUs. SBI-based computing, by contrast, could offer a much lower power draw for certain types of learning tasks—similar to how our own brains manage complex cognition using only about 20 watts.
Reference
YouTube
|
|